Research Supervision
Research Lead
• Full Professor, Youngjun Cho
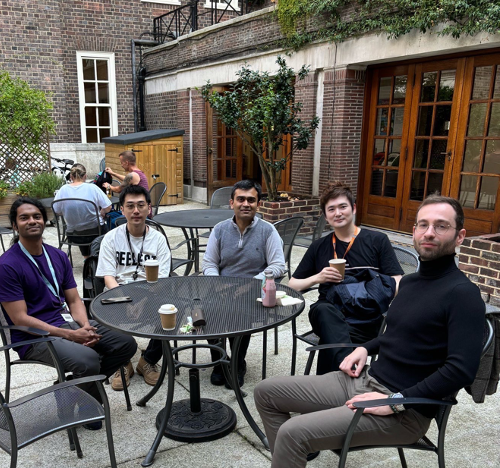
Research Fellows (Postdocs)
• Dr. Mark Quinlan (PhD in Explainable AI and Cyber Security from the University of Oxford)
• Dr. Farshid Rayhan (PhD in Machine Learning from the University of Manchester)
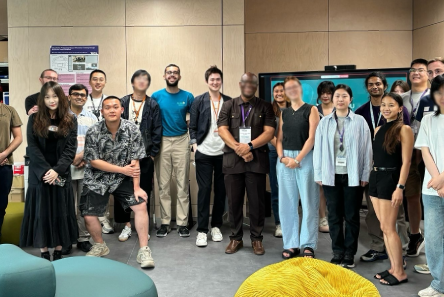
PhD students
• Jitesh Joshi (PhD student, Primary supervision)
• Katherine Wang (PhD student, Primary supervision)
• Zak Morgan (PhD student, Primary supervision)
• Merlin Kelly (PhD student, Primary supervision)
• Zikun Quan (PhD student, Primary supervision)
• Ziyue Zhu (PhD student, Co-supervision with Prof. Carlson)
• Roxana Ramirez Herrera (PhD student, Co-supervision with Prof Holloway, Dr. Carlson)
• Shu Zhong (PhD student, Co-supervision with Prof Obrist)
• Simon Wei (PhD student, Co-supervision with Prof. Berthouze)
• Yifu Liu (PhD student, Co-supervision with Prof. Berthouze)
• Alexander Thomas (PhD student, Co-supervision with Prof. Carlson)
We are now hiring PhD students !
Alumni (To be updated)
[Alumni – primary supervision only]
• Jade Savage (Physiological Computing, 2017/18 MSc in CSML, BSc from UCL) – DeepMind Scholarship | Microfocus
• Delaney Warren (Physiological Computing & AT, 2019/20 MSc in DDI, BSc from UCLA) – UCLFAA Scholarship
• Meg Obata (Physiological Computing & AT, 2019/20 MSc in DDI, BSc from Johns Hopkins University) – UCLFAA Scholarship
• Amit Patel (Machine Learning, 2018/19 MSc in ML)
• Chang Liu (Physiological Computing, 2018/19 MSc in DS) – Currently PhD Student
• Alok Suresh (Machine Learning, 2019/20 MSc in CSML) – Lanterne
• Zak Morgan (Machine Learning, 2019/20 MEng in CS, BSc from UCL) – Currently PhD Student
• Katherine Wang (Virtual Reality & AT, 2019/20 MSc in DDI, BSc from Penn state university) – Currently PhD Student
• James Wong-You (Human-Computer Interaction, 2018/19 MSc in HCI) – NHS
• Brian Min (Machine Learning, 2019/20 MEng in CS) – American Express
• Seunghoi Kim (Machine Learning, 2019/20 MEng in CS)
• Mahdi Nasrollahi (Machine Learning, 2019/20 MEng in CS)
• Su Yeon Oh (Haptics & AT, 2019/20 MSc in DDI)
• Yuliang Chen (Physiological Computing & AT, 2019/20 MSc in DDI)
• Bei Xiang (Haptics and Visual Impairment, 2019/20 MSc in DDI)
• Yu-Wei Yang (Physiological Computing and AT, 2020/21 MSc in DDI) – NHS
• Clara Moge (Physiological Computing, 2020/21 MSc in HCI, BSc from UCL) – Google / Youtube
• Yun Jung Yeh (Physiological Computing & AT, 2020/21 MSc in DDI) – JP Morgan
• William Davies (Physiological Computing, 2020/21 MSc in Machine Learning, BSc from UCL) – Amazon
• Steven McDonald (Physiological Computing, 2020/21 MSc in Machine Learning, BSc from Univ of Manchester) – Precise TV
• Yuxuan Liu (Physiological Computing & AT, 2020/21 MSc in DDI) – Huawei
• Pauline Hohl (Physiological Computing & AT, 2020/21 MSc in DDI) – CareLineLive
• Tianyun Hu (Physiological Computing, 2020/21 MSc in HCI) – Huawei
• Selina He (Physiological Computing, 2021/22 MSc in DDI, BSc from UCL) – KPMG
• Phoenix Wang (Physiological Computing, 2021/22 MSc in DDI, BSc from Univ of Toronto) – Cisco
• Sebastian Pauwels (Blockchain and Disability, 2021/22 MSc in DDI)
• Henry Drake (Physiological Computing, 2020-22 MSc in HCI, BSc from UCL)
• Sweccha Kansakar (Physiological Computing, 2018-22 BSc/MEng in CS) – Microsoft
• Rafaela Baquero Aguilar (Physiological Computing & VR, 2021/22 MSc in DDI, BSc from University of California, Berkeley)
• Rory Nicholas (Machine Learning, 2022/23 BSc in CS)
• Vincent Leong (Research Assistant in Bentley Comfort AI, 2021)
• Emil Almazov (Research Assistant in Bentley Comfort AI, 2021)
• Dr. Irena Arslanova (Short-term Postdoc, 2022)
• Yukun Zhou (Research Assistant in AI in Dementia Care, 2021-2022)
• Dr. Guangyu Ren (PhD in Deep Learning and Computer Vision from Imperial College London)
We are now hiring PhD students !
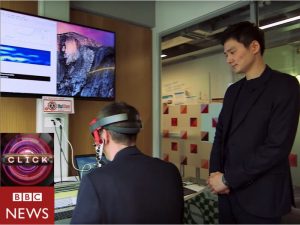
Non-contact Physiological Measurement
As humans are homeothermic, our internal temperature is closely linked with numerous physiological and psychological mechanisms. Given this, human thermal patterns have been explored to improve the understandings of our body for a couple of centuries (Cho, 2019). Researchers have shown that physiological signatures can be captured via non-contact thermal imaging (e.g. respiration monitoring in Cho et al. 2017). Whilst other contactless sensing methods, such as, RGB camera-based photo-plethysmography suffer from illumination and privacy issues, thermography is much less affected by those constraints (Lloyd, 2013). In addition, studies have shown that different types of physiological activities can be read through the use of a thermal imaging channel (Garbey et al., 2007, Pavlidis et al., 2012, Cho et al. 2017-2019), including respiratory, cardiovascular, perspiratory responses.
In our lab, we have been mainly investigating how to improve reliability, accuracy in physiological measurements through thermal imaging (to support real-world situations). At the same time, we have been building remote PPG (photo-plethysmography) algorithms using normal RGB cameras.
References
1. Cho, Y., Julier, S. J. & Bianchi-Berthouze, N. Instant Stress: Detection of Perceived Mental Stress Through Smartphone Photoplethysmography and Thermal Imaging. JMIR Mental Health 6, e10140 (2019).
2. Cho, Y., Julier, S. J., Marquardt, N. & Bianchi-Berthouze, N. Robust tracking of respiratory rate in high-dynamic range scenes using mobile thermal imaging. Biomed. Opt. Express, BOE 8, 4480–4503 (2017).
3. Cho, Y. Automated Mental Stress Recognition through Mobile Thermal Imaging. in the 7th International Conference on Affective Computing and Intelligent Interaction, ACII 2017 596–600 (2017).
4. Garbey, M., Sun, N., Merla, A. & Pavlidis, I. Contact-Free Measurement of Cardiac Pulse Based on the Analysis of Thermal Imagery. IEEE Transactions on Biomedical Engineering 54, 1418–1426 (2007).
5. Pavlidis, I. et al. Fast by Nature – How Stress Patterns Define Human Experience and Performance in Dexterous Tasks. Sci Rep 2, (2012).
6. Lloyd, J. M. Thermal Imaging Systems. (Springer Science & Business Media, 2013).
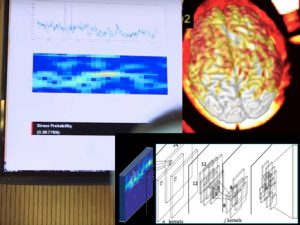
Automatic Recognition of Psychological States (eg. Mental Stress)
With sensing technology becoming pervasive in our everyday life, the ability to monitor human psychological states has become important in human computer interaction. Amongst such states, high level mental stress or mental workload is a common problem affecting mental, physical health and life in our modern society (Nash & Thebarge, 2006; McEwen, 2007; Arnsten, 2009). Studies show that mental stress could be automatically assessed through the use of these physiological sensing technologies, in turn opening new potential ways for stress management support strategies (Healey & Picard, 2005; Hosseini & Khalilzadeh, 2010; Hernandez et al., 2011; Hong et al., 2012; Sano & Picard, 2013; Al-Shargie et al., 2016; Yu et al., 2018; Cho et al., 2017,2018,2019). However, to date, such available technologies are still relatively fragile, or restrict mobility, movement and measurement environments, limiting their use. Given this we have been focusing on building new approaches to more reliable automatic recognition of psychological states, especially, mental stress, using low-cost and mobile sensing technology, supporting unconstrained and potentially a variety of everyday situations.
References
1. Nash, J. M. & Thebarge, R. W. Understanding Psychological Stress, Its Biological Processes, and Impact on Primary Headache. Headache: The Journal of Head and Face Pain 46, 1377–1386 (2006).
2. McEwen, B. S. Physiology and Neurobiology of Stress and Adaptation: Central Role of the Brain. Physiological Reviews 87, 873–904 (2007).
3. Arnsten, A. F. T. Stress signalling pathways that impair prefrontal cortex structure and function. Nat Rev Neurosci 10, 410–422 (2009).
4. Healey, J. A. & Picard, R. W. Detecting stress during real-world driving tasks using physiological sensors. IEEE Transactions on Intelligent Transportation Systems 6, 156–166 (2005).
5. Hosseini, S. A. & Khalilzadeh, M. A. Emotional Stress Recognition System Using EEG and Psychophysiological Signals: Using New Labelling Process of EEG Signals in Emotional Stress State. in 2010 International Conference on Biomedical Engineering and Computer Science 1–6 (2010). doi:10.1109/ICBECS.2010.5462520
6. Hernandez, J., Morris, R. R. & Picard, R. W. Call Center Stress Recognition with Person-Specific Models. in Affective Computing and Intelligent Interaction 125–134 (Springer, Berlin, Heidelberg, 2011). doi:10.1007/978-3-642-24600-5_16
7. Hong, J.-H., Ramos, J. & Dey, A. K. Understanding Physiological Responses to Stressors During Physical Activity. in Proceedings of the 2012 ACM Conference on Ubiquitous Computing 270–279 (ACM, 2012). doi:10.1145/2370216.2370260
8. Sano, A. & Picard, R. W. Stress Recognition Using Wearable Sensors and Mobile Phones. in 2013 Humaine Association Conference on Affective Computing and Intelligent Interaction 671–676 (2013). doi:10.1109/ACII.2013.117
9. Al-Shargie, F. et al. Mental stress assessment using simultaneous measurement of EEG and fNIRS. Biomedical optics express 7, 3882–3898 (2016).
10. Yu, B., Funk, M., Hu, J., Wang, Q. & Feijs, L. Biofeedback for Everyday Stress Management: A Systematic Review. Front. ICT 5, (2018).
11. Cho, Y. Automated Mental Stress Recognition through Mobile Thermal Imaging. in the 7th International Conference on Affective Computing and Intelligent Interaction, ACII 2017 596–600 (2017).
12. Cho, Y., Bianchi-Berthouze, N. & Julier, S. J. DeepBreath: Deep Learning of Breathing Patterns for Automatic Stress Recognition using Low-Cost Thermal Imaging in Unconstrained Settings. in the 7th International Conference on Affective Computing and Intelligent Interaction, ACII 2017 456–463 (2017).
13. Cho, Y., Julier, S. J. & Bianchi-Berthouze, N. Instant Stress: Detection of Perceived Mental Stress Through Smartphone Photoplethysmography and Thermal Imaging. JMIR Mental Health 6, e10140 (2019).